Pachama has adopted a simple, transparent approach to quantifying baseline uncertainty.
Landowners receive carbon credits by protecting or restoring forests to increase carbon storage on their land. But a carbon project only benefits the climate if the landowner would not have protected or restored their land without carbon crediting. A project’s baseline estimates the expected change in forest carbon under a landowner’s business-as-usual activity without crediting.
The number of carbon credits a project receives each year is just the difference between the project and baseline change in forest carbon. As you might imagine, the baseline can have a large influence, often the largest, on the number of credits a project receives each year.
The widely reported examples of over-crediting in today’s carbon market largely stem from the market’s current rules for computing baselines. Though these rules vary by credit registry, nearly all permit a range of project-by-project assumptions that can wither under further scrutiny (West et al. 2020; Guizar‐Coutiño et al. 2022).
In a previous blog post and research brief, we described Pachama’s work developing an algorithmic baseline to standardize conservative baseline calculations and prevent over-crediting.
A key advantage of an algorithmic baseline, compared to today’s baselines, which are manually calculated in Excel spreadsheets, is their reproducibility. In other words, an algorithmic baseline can be calculated identically many times over, making it possible to quantify the baseline’s uncertainty.
In this post, we explain what baseline uncertainty is, how it can be straightforward to quantify with algorithmic baselines, and why quantifying baseline uncertainty is essential for high-quality, transparent, and scalable credit issuance.
What is baseline uncertainty?
Today’s carbon market assumes a project’s baseline is perfectly accurate when issuing credits. This is a significant weakness. Remember, a baseline is meant to estimate business-as-usual land use if a landowner had not enrolled in the carbon market. But there can be a range in possible business-as-usual scenarios, and baseline uncertainty reflects this fact.
Because baselines under current market rules are highly tailored to individual projects and rely on project-specific assumptions, there is generally no easy way to compute their uncertainty.
An algorithmic baseline, however, applies a standardized set of calculations to input satellite data. Using the same input data, we can run the baseline algorithm tens or hundreds of times to compute the range in baseline outcomes across areas not enrolled in the carbon market.
How does Pachama quantify baseline uncertainty?
Pachama sees transparency in crediting as absolutely essential. Transparency means demystifying crediting, so that both landowners and buyers can easily understand how credit issuance is calculated. This is why Pachama has adopted a simple, straightforward approach to quantifying baseline uncertainty.
This approach uses pseudo projects, which are just randomly selected areas without a carbon project (Fig. 1). You can think of them as fake carbon projects or business-as-usual forests.
Pachama’s dynamic control area baseline algorithmically (see our explainer) selects control areas that match the carbon project across a variety of satellite observations. These control areas are just like the control group in any scientific experiment or pharmaceutical trial.
But how do we know if the selected control area is accurate?
We apply the baseline algorithm to each pseudo project. In other words, we select a control area for each pseudo project. We call this control area a pseudo control. The important thing to remember is that both the pseudo project and its pseudo control have no carbon project. So their outcomes should match (Fig. 2a) 1.
But no baseline algorithm is perfect. Some discrepancies between pseudo projects and their baselines (i.e., pseudo control areas) are inevitable. This is baseline uncertainty.
The best way to understand this is with an example. Imagine we randomly select 30 pseudo projects equal in size to a carbon project. Let’s say in one pseudo project we observe deforestation of 60,000 metric tons of carbon. But in its matching control area, we observe deforestation of 50,000 metric tons of carbon. So we know that when we match any project area to a control, the control area emissions (i.e., the baseline) can be off by as much as 10,000 metric tons, or 20% (10,000 ÷ 50,000).
Pachama uses these differences between pseudo projects and pseudo controls to compute a confidence band – a statistical upper and lower bound – around the project’s dynamic baseline. This confidence band captures the baseline’s annual uncertainty (Fig. 3.).
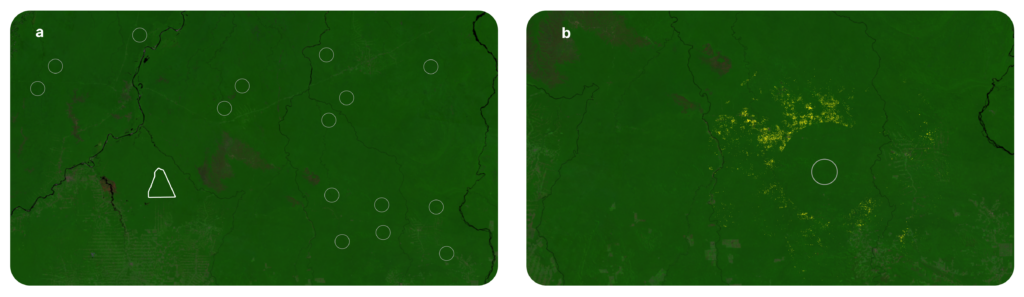
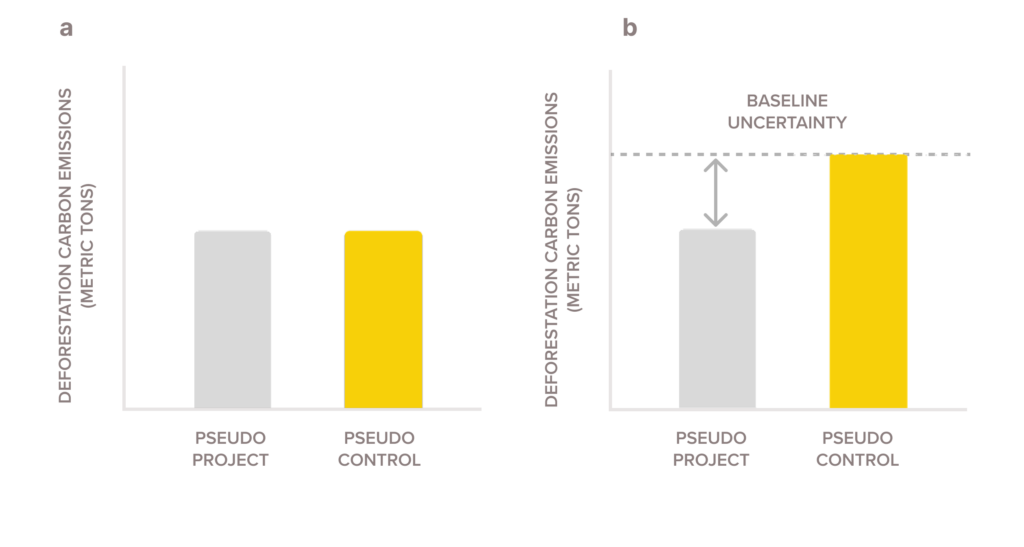
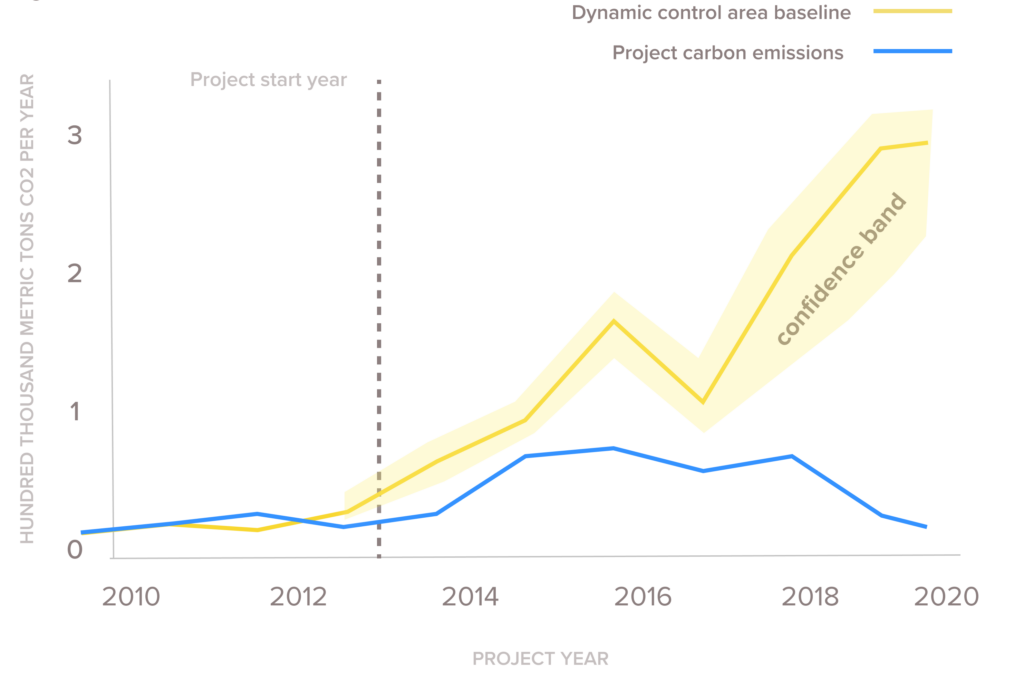
Why is quantifying baseline uncertainty crucial for high-quality, transparent, scalable carbon crediting?
The carbon market faces the challenge of growing to the scale needed to tackle climate change, while also strengthening credit quality and transparency to build trust. Crediting rules that account for baseline uncertainty can help advance all of these objectives.
- Quality: By quantifying baseline uncertainty, more conservative baselines can be used to ensure that each credit represents real climate impact.
- Transparency: Imagine you’re a credit buyer or a landowner looking to enroll in the carbon market. How do you know if a baseline is any good? Pseudo projects allow you to immediately know a baseline’s accuracy without having to grasp all of the technical details of how the baseline was calculated. The more accurate the baseline, the more outcomes between pseudo projects and their baselines will match (Fig. 3). Another major advantage of pseudo projects is that they can be used to quantify uncertainty for any algorithmic baseline, including the algorithms proposed for nesting project-level baselines within a jurisdiction-level baseline.
- Scale: the market faces the challenge of continually improving its rules to strengthen credit quality while preserving the integrity of past crediting. If the market adopts uncertainty-adjusted baselines, past baselines can remain valid, even as improving baseline algorithms continue to reduce uncertainty 2.
Pachama is encouraged to see a few credit registries moving to adopt more algorithmic baselines. These improvements to crediting rules could be made even stronger if they incorporated baseline uncertainty.
Subscribe to our newsletter to keep up-to-date about Pachama’s progress toward our vision of quality, transparent, and scalable crediting! We welcome your questions or feedback at [email protected]. For media inquiries please contact us at [email protected].
Footnotes
Pseudo-projects can be used to quantify uncertainty for any algorithmic baseline, including nested baselines. Nested baselines assign a fraction of a jurisdiction-level baseline to a project based on the project’s deforestation risk. By overlaying pseudo-projects on a historical risk map, the predicted historical deforestation for every pseudo-project can be calculated. This prediction is then compared to the past deforestation actually observed in the pseudo-project.
Past baselines are more likely to continually prove valid as baseline algorithms improve, if pseudo-projects show the baseline algorithm is unbiased. The past baseline would just be too conservative due to the higher uncertainty of earlier algorithm versions. For this reason, Pachama evaluates the bias of our dynamic control area baseline for each carbon project we examine.